How AI Risk Management Protects Customer Data
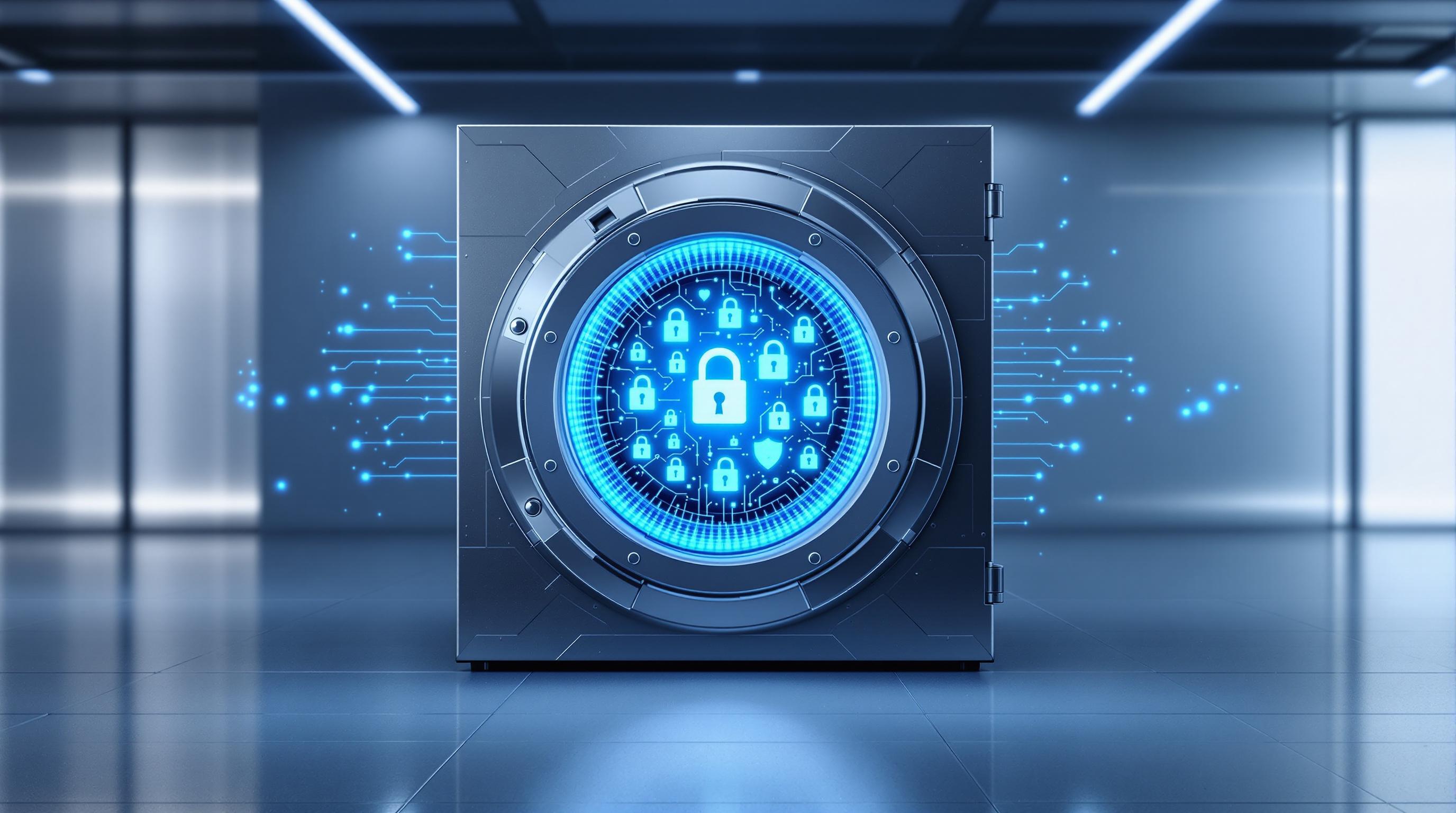
In this article
Table of Contents
- Understanding AI Risk Management: Key Concepts and Frameworks
- Identifying and Managing AI Risks
- Implementing AI Risk Management Strategies
- Setting Up Governance and Oversight
- Mitigating Risks with Data Privacy Protocols
- Complying with Industry Regulations
- Monitoring and Updating AI Risk Management Practices
- Practical Applications: AI Risk Management in Customer Service
- Protecting Customer Data with AI Risk Management
AI risk management is about keeping customer data safe while using AI systems. It helps businesses prevent data breaches, comply with laws like GDPR and CCPA, and maintain customer trust. Here’s a quick summary of how it works:
- Identify Risks: Spot vulnerabilities like data breaches, privacy issues, and biases in AI algorithms.
- Mitigate Risks: Use encryption, access controls, and real-time monitoring to secure data.
- Ensure Compliance: Follow regulations with regular audits and clear policies.
- Increase Transparency: Explain AI decisions to build trust and accountability.
Understanding AI Risk Management: Key Concepts and Frameworks
AI risk management is a critical part of protecting customer data in today's business world. It revolves around three main pillars: risk identification, mitigation, and compliance.
Using the NIST AI Risk Management Framework as a guide, here's how its components help safeguard customer data:
Framework Component | Purpose | Key Activities for Customer Protection |
---|---|---|
Governance | Establish oversight | Define accountability for data handling |
Mapping | Identify vulnerabilities | Track customer data touchpoints |
Measuring | Assess risk impact | Evaluate potential customer data exposure |
Managing | Implement controls | Deploy customer data safeguards |
One way this framework is applied is through real-time monitoring. AI systems can analyze patterns to detect security threats before they compromise customer data.
Success in this area depends on collaboration between executives, AI developers, data scientists, and compliance officers. Each plays a role in creating a thorough approach to protecting customer information.
Key areas organizations need to address include:
- Data Privacy Standards: Ensuring AI complies with regulations like GDPR and CCPA, particularly around transparency in algorithms
- Security Measures: Implementing strong encryption and access control systems
- Audit Trails: Keeping detailed records of AI decisions for accountability
AI also strengthens customer relationship management systems by improving data security through activities like:
Activity | Purpose |
---|---|
Risk Assessment | Evaluating complex scenarios affecting customer data |
Policy Development | Creating flexible security protocols |
Compliance Monitoring | Ensuring adherence to regulations |
Incident Response | Responding effectively to security breaches |
Regular updates to AI frameworks are essential to address emerging threats and technologies. While AI plays a significant role, human expertise is still necessary for context and making critical decisions. These principles lay the groundwork for businesses to tackle AI-related risks effectively.
Identifying and Managing AI Risks
Identifying Data Privacy and Security Risks
AI systems that handle customer data come with various vulnerabilities that demand attention. Key concerns include unauthorized access, data breaches, and biases in AI algorithms, all of which can threaten sensitive information.
To address these risks, real-time monitoring plays a critical role. This involves continuously scanning for threats and responding quickly. AI-powered tools help by analyzing patterns and detecting unusual activity, flagging potential problems before they escalate into major breaches.
Here are some common vulnerabilities in AI systems and how they can be detected:
Vulnerability Type | Risk Description | Detection Method |
---|---|---|
Phishing Attacks | Gaining access through deceptive tactics | AI pattern recognition |
Malware Infections | Breach caused by malicious code | Real-time monitoring |
Data Leakage | Exposure of sensitive information | Log analysis |
Algorithm Bias | Unfair processing of customer data | Regular audits |
Assessing and Prioritizing Risks
Risk assessment requires a mix of automated tools and human judgment. Predictive analytics help by examining historical patterns to predict potential threats, focusing on three main factors: the likelihood of the risk, its potential impact, and the time needed to recover.
The evaluation process considers these dimensions:
Assessment Factor | Evaluation Criteria | Impact on Protection |
---|---|---|
Risk Likelihood | Analyzing past incidents | Enables preventive measures |
Potential Impact | Level of sensitive data at risk | Guides response planning |
Recovery Time | Time to restore operations | Informs resource allocation |
To enhance security, AI-powered tools in CRM systems can:
- Block access from suspicious IP addresses
- Monitor account activity
- Flag unusual patterns for review
- Track and control data access
Combining regular security audits with AI-driven monitoring strengthens defenses against new threats. While AI excels at detecting risks quickly, human expertise is still essential for understanding context and making strategic decisions. After identifying and ranking risks, businesses should roll out targeted strategies to address them effectively.
Implementing AI Risk Management Strategies
Setting Up Governance and Oversight
Governance is crucial for managing risks in AI operations. It involves creating a structured approach with technical experts, data privacy specialists, and compliance officers working together. This team operates within defined frameworks and uses monitoring systems to ensure everything stays on track.
Here’s how governance is structured:
Component | Purpose | Implementation |
---|---|---|
Risk Management Team | Supervise AI operations | Assign a governance lead and recruit specialists |
Policy Framework | Direct AI usage | Develop guidelines for data collection, storage, and usage |
Audit System | Ensure compliance | Schedule regular reviews with clear escalation procedures |
Mitigating Risks with Data Privacy Protocols
Protecting customer data is a top priority, and this is where solid data privacy protocols come into play. Using advanced encryption and access controls, organizations can secure data during storage, transmission, and use - without disrupting efficiency.
Key data privacy measures include:
Protection Layer | Implementation | Security Benefit |
---|---|---|
Data Encryption | Use end-to-end encryption | Blocks unauthorized access to sensitive data |
Access Control | Apply multi-factor authentication | Restricts access to critical information |
Data Minimization | Collect only necessary data | Limits the impact of potential breaches |
Complying with Industry Regulations
Strong data privacy measures are important, but they must also align with legal requirements. Building compliance into AI systems from the start ensures adherence to industry standards.
To achieve this, organizations should:
- Perform regular audits
- Keep detailed records of AI decisions
- Create clear protocols for handling data requests
Monitoring and Updating AI Risk Management Practices
Regular Monitoring and Auditing
Keeping an eye on AI systems that handle customer data is essential for managing risks effectively. Organizations should use detection tools to spot vulnerabilities early, enabling quick action to prevent potential issues. Automated systems for real-time anomaly detection are crucial here.
By catching problems early, businesses can avoid data breaches that damage trust and expose sensitive information. Monitoring efforts should focus on three main areas:
Monitoring Area | Purpose | Implementation Method |
---|---|---|
System Behavior | Track how AI systems perform and handle data | Real-time AI analytics |
Access Patterns | Detect unauthorized access or unusual user behavior | Automated threat detection with behavioral analysis |
Data Flow | Follow the movement of customer data through systems | End-to-end tracking with anomaly detection |
In addition to ongoing monitoring, regular audits dig deeper into system security. These audits should examine both technical and compliance aspects, ensuring full protection. To stay ahead of new risks, businesses must also revise their strategies regularly.
Updating Strategies for New Risks and Technologies
As technology advances, so do security threats. Organizations need frameworks that can adjust to evolving risks and safeguard customer data effectively.
A structured plan for updating strategies might include:
Update Component | Frequency | Key Activities |
---|---|---|
Risk Assessment | Quarterly | Review emerging AI-related threats |
Policy Review | Bi-annual | Refresh governance and security protocols |
Technology Integration | Ongoing | Adopt new security tools and AI-driven defenses |
Cross-functional teams are vital in this process. Bringing together AI developers, data scientists, security experts, and compliance officers ensures risks are assessed and addressed from all angles.
Organizations should also create response plans for new threats while improving existing measures. This includes regular training for team members and keeping AI risk management practices up to date. These steps help businesses maintain both trust and compliance as they continue adopting AI solutions.
Practical Applications: AI Risk Management in Customer Service
Understanding AI risk management strategies is just the beginning. Now, let's see how these ideas come to life in customer service, where protecting sensitive data is a top priority.
Using AI for Safe Customer Communication
AI tools are now a mainstay in customer service, helping companies handle high volumes of inquiries while keeping data secure. To ensure these systems operate safely, businesses must prioritize security measures that protect sensitive information during AI-driven interactions.
Here are some key measures for secure AI-powered communication:
Security Measure | Implementation | Benefit |
---|---|---|
End-to-End Encryption | Secures data transmission between systems and users | Blocks unauthorized access to conversations |
Role-Based Access | Restricts AI systems to only necessary data | Minimizes chances of data exposure |
Real-Time Monitoring | Uses AI to detect unusual activity | Quickly flags potential security threats |
Striking the right balance between efficient customer service and strict data privacy measures is crucial. A great example of this is Dialzara, a platform that demonstrates how to prioritize security while leveraging AI.
Dialzara: A Case Study in Secure AI Use
Dialzara showcases how businesses can integrate AI tools without compromising on data security. The platform employs several standout practices to ensure customer data remains protected:
1. Secure Integration
Dialzara connects with more than 5,000 business applications, all while adhering to stringent data protection standards. This ensures customer information is safeguarded at every touchpoint.
2. Privacy-First Design
The platform’s AI voice technology includes advanced security features designed to protect sensitive information:
Feature | Security Benefit |
---|---|
Encrypted Communications and Storage | Keeps conversations and collected data safe |
Access Control Systems | Prevents unauthorized access to sensitive information |
3. Compliance and Monitoring
Dialzara ensures it meets data protection regulations while operating 24/7. Regular system audits and updates bolster its security, aligning with UiPath's emphasis on trust, transparency, and control.
Protecting Customer Data with AI Risk Management
In customer service examples like Dialzara, managing AI-related risks plays a key role in keeping sensitive data safe. By using frameworks like NIST and applying practical approaches, businesses can build strong systems to protect customer information.
Strong AI risk management combines advanced tools, clear procedures, and skilled teams. When done right, it offers three main advantages:
Benefit | Impact | Outcome |
---|---|---|
Preventing Risks Early | Detecting threats quickly | Fewer data breaches |
Meeting Regulations | Automated compliance checks | Consistent GDPR/CCPA compliance |
Building Customer Trust | Clear AI practices | Better customer relationships |
To stay ahead of new threats, companies should focus on regular audits, updating policies, and ongoing training. As AI continues to develop, it's crucial to prioritize clear governance and strong data privacy measures. This also means ensuring AI decisions are fair and being transparent about how customer data is used and protected.