10 Common Ethical Issues in AI & Solutions
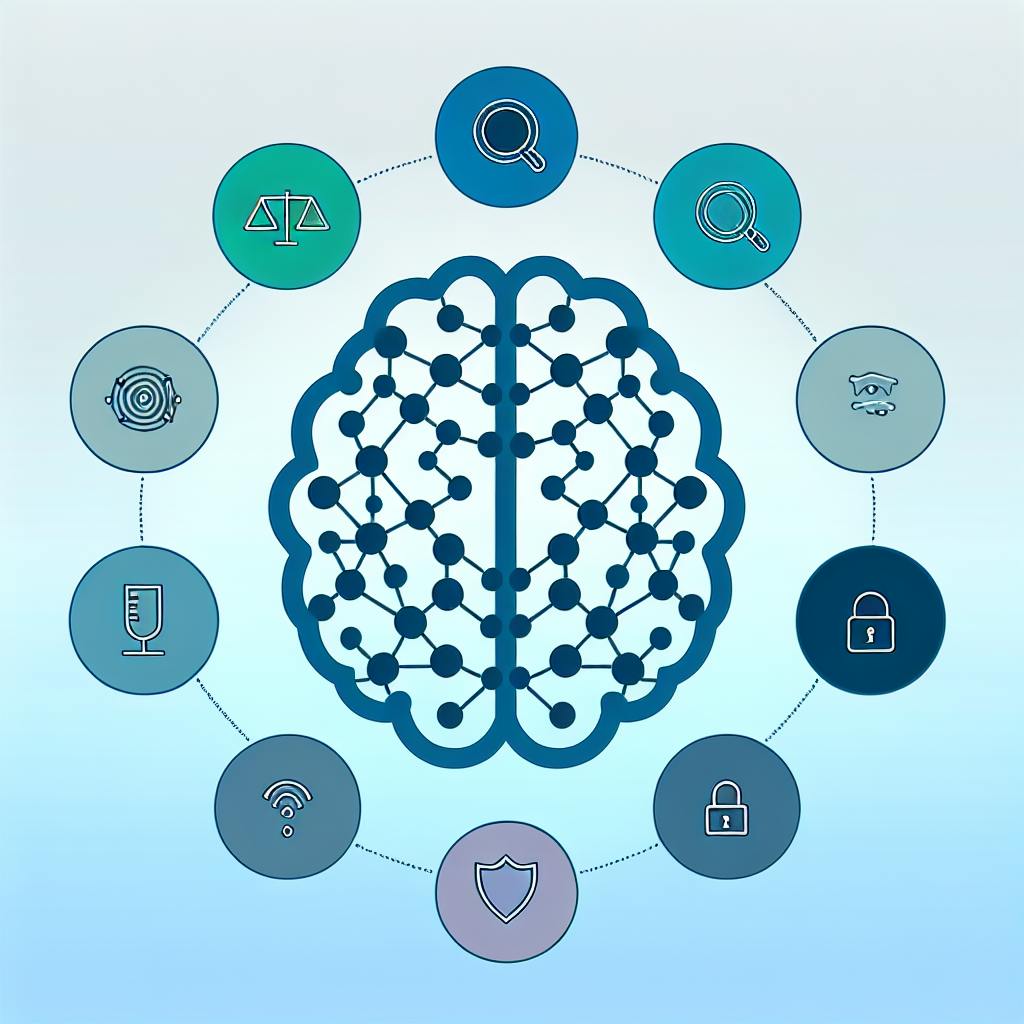
Explore the common ethical issues in AI, such as bias, lack of transparency, privacy risks, safety concerns, and trust issues. Learn about potential solutions and how to address these challenges.
10 Common Ethical Issues in AI & Solutions
As AI becomes more prevalent, addressing ethical concerns is crucial. Here are the key issues and potential solutions:
Ethical Issues
- Bias and Discrimination: AI can perpetuate societal biases, leading to unfair treatment based on factors like race, gender, or economic status.
- Privacy Risks: AI systems process large amounts of personal data, raising privacy concerns if not handled properly.
- Lack of Transparency: Many AI systems operate as "black boxes," making it difficult to understand how they make decisions.
- Safety Concerns: Ensuring AI operates safely and reliably, minimizing risks of harm or unintended consequences.
- Lack of Explainability: Users must understand how AI systems reach conclusions, especially in high-stakes domains.
- Need for Human Oversight: Human involvement is key to ensuring AI works properly, ethically, and effectively.
- Trust Issues: Users need to feel confident that AI systems are ethical, reliable, and fair.
- Job Displacement: AI automation could potentially displace human workers, leading to job losses.
- Cybersecurity Threats: AI systems can be vulnerable to cyber attacks, data breaches, and other security threats.
- Long-term Impact: Considering AI's effects on the environment, jobs, and society in the long run.
Potential Solutions
- Implement ethical AI frameworks and guidelines
- Promote diversity and inclusivity in AI development
- Prioritize transparency and explainability
- Conduct rigorous testing and auditing for bias and fairness
- Establish clear governance and accountability measures
- Invest in responsible AI research and development
- Collaborate with policymakers and stakeholders
- Educate and train employees on AI ethics
- Adopt ethical AI principles and best practices
- Continuously monitor and evaluate AI systems for ethical compliance
Issue | Impact | Feasibility | Urgency |
---|---|---|---|
Unfair Bias | High | Medium | High |
Lack of Transparency | Medium | High | Medium |
Privacy Risks | High | Medium | High |
Safety Concerns | High | High | High |
Lack of Explainability | Medium | Medium | Medium |
Need for Human Oversight | High | Medium | High |
Trust Issues | High | Medium | High |
Job Displacement | High | Medium | High |
Cybersecurity Threats | High | High | High |
Long-term Societal Impact | High | Medium | High |
Addressing ethical issues in AI is crucial for responsible development and deployment. Businesses must take proactive steps to ensure fair, transparent, and trustworthy AI systems that benefit society.
Related video from YouTube
1. Bias and Unfairness
AI systems can show bias and unfairness due to issues with the data used to train them. This can lead to unfair treatment based on factors like race, gender, age, or economic status.
Types of Bias
1. Historical Bias
The existing biases in society can get encoded into the data used to train AI models.
2. Representation Bias
How we define and sample populations to create datasets can lead to under- or over-representation of certain groups.
3. Measurement Bias
The choice of features or labels used in predictive models can introduce bias.
Solutions
To address bias and unfairness, businesses can take these steps:
- Diverse Data: Collect data from various sources, locations, demographics, and backgrounds to ensure diversity and representation.
- Debiasing Techniques: Use methods like data augmentation to reduce bias in models.
- Regular Audits: Conduct regular testing to identify and mitigate bias in AI systems.
- Transparency: Prioritize transparency and explainability in AI systems, using techniques like model interpretability to understand how predictions are made.
- Governance: Establish clear governance and accountability measures to ensure fairness and unbiased AI systems.
Solution | Description |
---|---|
Diverse Data | Collect data from various sources to ensure diversity and representation. |
Debiasing Techniques | Use methods like data augmentation to reduce bias in models. |
Regular Audits | Conduct regular testing to identify and mitigate bias. |
Transparency | Prioritize transparency and explainability in AI systems. |
Governance | Establish clear governance and accountability measures. |
Other practical steps include:
- Implementing ethical AI guidelines
- Promoting diversity in AI development teams
- Investing in responsible AI research and development
2. Transparency
Transparency is vital for AI systems, as it allows users to understand how these systems make decisions and ensures accountability. AI transparency means making artificial intelligence systems understandable and interpretable to humans. This "open book" approach lets users comprehend the logic, functions, and decision-making processes of AI systems.
Without transparency, AI systems can seem like "black boxes" where the decision-making process is unclear, leading to a lack of trust and potential biases. Transparency in AI ensures that users can identify biases, errors, and inconsistencies, enabling them to take corrective action.
The benefits of AI transparency include:
Benefit | Description |
---|---|
Building Trust | Transparency builds trust with customers and employees by providing a clear understanding of AI decision-making processes. |
Ensuring Fairness | Transparency ensures fair and ethical AI systems by detecting and addressing biases. |
Identifying Biases | Transparency detects and addresses potential data biases, ensuring accurate and reliable AI systems. |
Improving Performance | Transparency enhances the accuracy and performance of AI systems by identifying errors and inconsistencies. |
Ensuring Compliance | Transparency ensures compliance with regulations, reducing the risk of legal and reputational damage. |
3. Privacy
AI systems can process large amounts of personal data, which raises privacy concerns if not handled properly.
Protecting Personal Data
AI systems must have strong security measures to prevent unauthorized access to personal data. This includes:
- Encryption
- Access controls
- Secure data storage
AI systems must also follow data protection laws like the GDPR in the EU.
Transparency and Consent
AI systems should be clear about:
- What personal data they collect
- How the data is used
- Who the data is shared with
People must give informed consent before their data is collected. They should also have the right to withdraw consent and access or delete their personal data.
Anonymizing and Pseudonymizing Data
AI systems can use these techniques to protect personal data:
Technique | Description |
---|---|
Anonymization | Removing identifying information from data. |
Pseudonymization | Replacing identifying information with an artificial ID. |
These techniques allow AI systems to process data while protecting individual privacy.
4. Safety
Ensuring AI systems operate safely and reliably is crucial, minimizing risks of harm or unintended consequences. As AI becomes more autonomous and integrated into various aspects of life, the potential risks of accidents, errors, or misuse increase.
Establishing Clear Standards
To mitigate these risks, it's essential to establish clear safety standards and guidelines for AI development, deployment, and use. This includes:
- Defining acceptable behavior and performance levels
- Conducting rigorous testing to identify potential vulnerabilities
- Implementing secure and transparent AI systems for easy monitoring and correction
- Ensuring accountability in AI development and deployment
Limiting Access and Privileges
Another key aspect is limiting access and privileges to AI systems. This involves:
Action | Description |
---|---|
Limiting Access | Restricting access to sensitive data and systems |
Authentication | Implementing robust authentication mechanisms |
Authorization | Ensuring AI systems operate within predetermined boundaries |
Human Oversight and Intervention
Human oversight and intervention are critical components:
- Mechanisms for human intervention if AI systems fail or malfunction
- Ability for humans to correct or shut down AI systems if necessary
- Developing AI systems that can explain their decision-making processes
5. Explainability: Understanding AI Decisions
Explainability allows users to comprehend how AI systems reach their conclusions. This is crucial in areas like healthcare, finance, and law, where AI decisions can significantly impact people's lives. Explainability builds trust, transparency, and accountability, enabling users to identify biases, errors, and inconsistencies.
Techniques for Clarity
Several methods can make AI systems more understandable:
- Model interpretability: Designing AI models that provide insights into their decision-making processes. For example, feature importance analysis, partial dependence plots, and SHAP values can explain how AI models make predictions.
- Model-agnostic explanations: Explaining AI models without modifying their architecture. Techniques like LIME (Local Interpretable Model-agnostic Explanations) and TreeExplainer can generate explanations for any machine learning model.
- Transparency in development: Providing clear documentation and logging of AI development processes, including data sourcing, model training, and testing. This transparency helps users understand how AI systems are designed and trained.
Benefits of Explainability
Explainability in AI offers several advantages:
Benefit | Description |
---|---|
Trust | When users understand how AI systems reach conclusions, they are more likely to trust the results. |
Identifying Issues | Explainability helps identify biases and errors in AI systems, enabling developers to correct them. |
Accountability | Explainability ensures that AI systems are accountable for their decisions, reducing the risk of unintended consequences. |
6. Human Oversight
Having people involved in the process is key to ensuring AI systems work properly, ethically, and effectively. This means having humans review, make decisions, and monitor AI systems. Here are some practical ways to include human oversight when using AI:
Set Clear Roles and Responsibilities
Decide who will be responsible for:
- Reviewing AI-generated content
- Making decisions on questionable outputs
- Addressing ethical concerns
Include Human Review Steps
Have people review AI outputs, especially for sensitive topics like:
- Health recommendations
- Legal advice
- Areas where incorrect information could cause harm
Create Ethical Guidelines
Develop a set of ethical standards and best practices for using AI in your organization. These should cover issues like:
- Bias
- Privacy
- Accuracy of AI-generated content
Train all human reviewers on these guidelines.
Use Hybrid Decision-Making
Combine AI and human decision-making. For example:
- AI handles routine tasks or creates initial drafts
- Humans refine, verify, or approve the final output
This ensures the highest quality standards.
Ongoing Training
Provide regular training for those involved in human oversight, including:
- Understanding AI capabilities and limitations
- Staying updated on ethical AI practices
- Learning how to effectively review and improve AI outputs
7. Trustworthiness
Building trust is crucial for AI systems. Users need to feel confident that these systems are ethical, reliable, and fair. Trustworthy AI systems:
- Are transparent about how they work and make decisions
- Respect human values and rights
- Follow ethical standards
- Provide consistent and dependable outputs
To promote trust, businesses should:
Explain AI Decisions
Users must understand how AI systems reach conclusions. Clear explanations make the systems more accountable and reliable.
Ensure Fairness
AI systems must be unbiased and treat everyone fairly, regardless of factors like race, gender, or economic status.
Establish Responsibility
There should be clear lines of responsibility for AI decision-making. Users need to know who is accountable.
Provide Access
Give users access to their data and explanations for AI-driven decisions. This openness builds confidence.
Key Points
Action | Purpose |
---|---|
Explain Decisions | Make AI systems understandable and accountable |
Ensure Fairness | Prevent biases and unfair treatment |
Establish Responsibility | Clarify who is accountable for AI decisions |
Provide Access | Allow users to view their data and AI explanations |
8. Job Displacement
As AI systems become more capable, they may automate certain tasks, potentially replacing human workers. This could lead to job losses, especially in industries with repetitive or easily automated tasks.
To address this concern, businesses should consider:
Provide Training and Development
Invest in programs to help employees acquire new skills related to AI, such as data analysis and machine learning. This will enable them to adapt to changing job requirements.
Encourage Innovation
While AI may replace some jobs, it can also create new opportunities. Promote innovation and entrepreneurship in AI-related fields like development, deployment, and maintenance.
Offer Job Transition Support
Develop programs to assist workers in transitioning to new roles or industries. This can involve partnerships with educational institutions and government agencies to provide training and support.
Key Points
Action | Purpose |
---|---|
Provide Training and Development | Help employees acquire new AI-related skills |
Encourage Innovation | Create new job opportunities in AI-related fields |
Offer Job Transition Support | Assist workers in transitioning to new roles or industries |
9. Cybersecurity
AI systems can be vulnerable to cyber attacks, data breaches, and other security threats. This can lead to major problems like financial losses, damage to reputation, and sensitive information being compromised.
To address cybersecurity concerns, businesses should take these steps:
Implement Strong Security Measures
Set up a solid security foundation by using strong security measures like:
- Encryption
- Firewalls
- Intrusion detection systems
Regularly update and patch AI systems to fix any security weaknesses.
Conduct Regular Security Checks
Perform regular security audits to find potential vulnerabilities and weaknesses in AI systems. This helps detect and respond to security threats before they cause harm.
Develop Response Plans
Have plans ready to quickly respond to security breaches or incidents. This ensures businesses can minimize damage and restore systems quickly.
Action | Purpose |
---|---|
Implement Strong Security Measures | Prevent cyber attacks and data breaches |
Conduct Regular Security Checks | Identify and address vulnerabilities |
Develop Response Plans | Quickly respond to security incidents |
10. Long-term Impact
As AI systems grow more advanced and widespread, it's crucial to consider their long-term effects on the environment, jobs, and society.
Environmental Concerns
AI systems require immense energy for production and operation, contributing to greenhouse gas emissions and climate change. Training large AI models is highly energy-intensive, leaving a substantial carbon footprint. Additionally, the disposal of AI hardware and mining of rare metals used in production can severely damage the environment.
To reduce AI's environmental impact:
- Develop more energy-efficient AI systems
- Prioritize sustainability in AI design and deployment
Job Displacement and Unintended Effects
While AI can automate tasks and boost productivity, it also risks displacing workers and worsening social inequalities. AI systems can also have unintended consequences, like perpetuating biases and discrimination, with far-reaching negative impacts.
To address these issues:
Action | Purpose |
---|---|
Develop transparent and explainable AI | Ensure AI systems are fair and understandable |
Prioritize social benefits | Ensure AI technologies benefit all members of society |
Key Points
- AI systems' energy demands contribute to climate change
- Sustainable and energy-efficient AI is crucial
- Job displacement and social inequalities are risks
- Transparent, fair, and socially beneficial AI is essential
- Unintended consequences must be mitigated
Comparing Ethical Issues in AI
Issue | Impact | Feasibility | Urgency |
---|---|---|---|
Unfair Bias | High | Medium | High |
Lack of Transparency | Medium | High | Medium |
Privacy Risks | High | Medium | High |
Safety Concerns | High | High | High |
Lack of Explainability | Medium | Medium | Medium |
Need for Human Oversight | High | Medium | High |
Trust Issues | High | Medium | High |
Job Displacement | High | Medium | High |
Cybersecurity Threats | High | High | High |
Long-term Societal Impact | High | Medium | High |
Unfair bias in AI can lead to discrimination, perpetuating inequalities. Addressing bias is crucial but complex due to AI algorithms and the need for diverse data. While mitigating bias is possible through techniques like data debiasing and algorithm adjustments, it requires significant effort and resources, making it a medium feasibility task.
Lack of transparency in how AI makes decisions raises concerns about accountability and trust. Ensuring transparency is highly feasible through techniques like model interpretability and explainable AI. However, balancing transparency with protecting proprietary algorithms and data can be challenging, making it a medium urgency issue.
Privacy risks arise from the vast personal data used to train AI models. Addressing privacy through data anonymization, encryption, and regulatory compliance is feasible but requires significant effort, making it a medium feasibility task. Given potential privacy violations and growing public awareness, this issue has high urgency.
Safety concerns related to AI systems, such as autonomous vehicles or robots, are of high urgency due to potential physical harm. Ensuring safety through rigorous testing, failsafe mechanisms, and human oversight is highly feasible but requires substantial resources and effort, making it a high feasibility task.
Lack of explainability in how AI makes decisions can hinder trust and accountability. While techniques like model interpretability and explainable AI can improve explainability, their effectiveness and feasibility vary based on the AI system's complexity, making it a medium feasibility and urgency issue.
The need for human oversight and control over AI systems is crucial to mitigate risks and ensure ethical behavior. While human oversight is feasible through techniques like human-in-the-loop and human-on-the-loop strategies, it requires significant resources and can potentially limit AI capabilities, making it a medium feasibility task with high urgency.
Trust issues in AI systems can arise from various factors, including lack of transparency, accountability, and potential for unfair or harmful outcomes. Building trust is a medium feasibility task that requires addressing multiple ethical concerns, such as bias, privacy, and safety, making it a high urgency issue.
Job displacement due to AI automation is a high impact concern, as it can lead to widespread unemployment and societal disruption. Mitigating these risks through workforce retraining, job creation, and responsible AI deployment is a medium feasibility task that requires significant effort and resources, making it a high urgency issue.
Cybersecurity threats to AI systems, such as adversarial attacks or data poisoning, can compromise the integrity and safety of these systems. Ensuring robust cybersecurity measures is highly feasible but requires substantial resources and effort, making it a high feasibility and urgency task.
The long-term societal impact of AI systems, including environmental concerns, job displacement, and unintended consequences, is a high impact issue. Mitigating these risks through sustainable AI development, responsible deployment, and proactive measures is a medium feasibility task that requires significant effort and resources, making it a high urgency concern.
Conclusion
Addressing ethical issues in AI is crucial as AI becomes more widespread. Business owners and managers must take proactive steps to ensure responsible AI development and use. The 10 common ethical issues discussed in this article, including bias, lack of transparency, privacy risks, safety concerns, lack of explainability, need for human oversight, trust issues, job displacement, cybersecurity threats, and long-term societal impact, highlight the importance of a comprehensive approach to AI ethics.
To ensure ethical AI usage, businesses should:
1. Develop an AI ethics code
Establish clear guidelines and principles for responsible AI development and deployment.
2. Ensure diversity and inclusion
Promote diversity in AI development teams to mitigate biases and ensure fair representation.
3. Monitor AI systems
Continuously monitor AI systems for potential issues, such as bias, privacy violations, or safety concerns.
4. Educate employees
Provide training to employees on AI ethics, responsible use, and potential risks.
5. Be transparent
Maintain transparency about how AI systems work, their decision-making processes, and the data used to train them.
6. Address privacy concerns
Implement robust data protection measures, obtain consent, and anonymize or pseudonymize personal data when necessary.
7. Consider human rights
Ensure AI systems respect human rights and do not infringe on individual freedoms or perpetuate discrimination.
8. Anticipate risks
Proactively identify and mitigate potential risks, such as job displacement, cybersecurity threats, and environmental impact.
9. Conduct ethical reviews
Regularly review AI systems and processes to ensure compliance with ethical standards and address any concerns.
10. Partner with ethical providers
Work with AI providers and vendors that prioritize ethical AI development and adhere to responsible practices.
FAQs
What are the ethical considerations for AI design?
When creating AI systems, it's crucial to ensure they are fair and unbiased. This means taking steps to prevent discrimination based on factors like race, gender, or economic status. It also involves carefully examining the data used to train the AI system. If the training data contains biases, the AI will likely produce biased results.
AI designers must also consider the potential impact of their creations on society. This includes thinking about how AI systems might reinforce existing inequalities or create new ones. By being aware of these risks, designers can work to mitigate them and create more equitable AI systems.
Overall, the ethical considerations for AI design are complex and require careful thought and attention to detail. The goal is to create AI that benefits society as a whole.
Key Points
Consideration | Description |
---|---|
Fairness and Bias | Prevent discrimination based on factors like race, gender, or economic status. Ensure training data is diverse and unbiased. |
Societal Impact | Consider how AI systems might reinforce or create new inequalities. Work to mitigate these risks. |
Careful Design | Ethical AI design requires complex thought and attention to detail. The goal is to create AI that benefits society. |
Ready to Transform Your Phone System?
See how Dialzara's AI receptionist can help your business never miss another call.