AI Transparency & Accountability: Principles, Differences
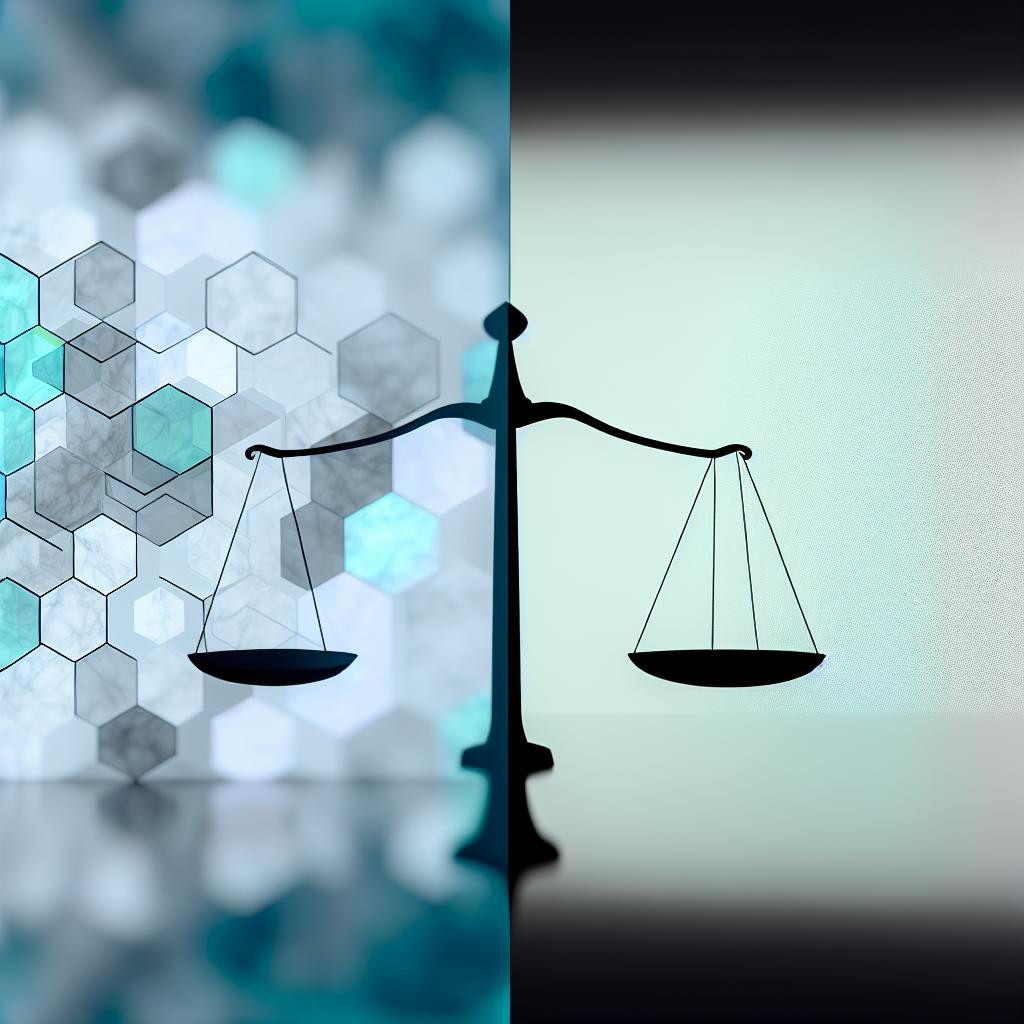
Learn about the principles, differences, benefits, and challenges of AI transparency and accountability in this detailed article. Explore key components and understand the importance of responsible AI development.
AI Transparency & Accountability: Principles, Differences
AI transparency means making the decisions and processes of AI systems clear and understandable. AI accountability involves holding organizations and individuals responsible for the actions and impacts of their AI systems.
AI Transparency
- Ensures AI decisions are fair, unbiased, and reliable
- Builds trust in AI by explaining how it works
- Helps identify and prevent biases
AI Accountability
- Promotes ethical and responsible development of AI
- Ensures organizations act responsibly with AI
- Helps avoid unintended harm from AI systems
Key Differences
Aspect | AI Transparency | AI Accountability |
---|---|---|
Definition | Understanding AI decisions | Responsibility for AI outcomes |
Focus | Clarity and fairness | Ethical use |
Importance | Builds trust, prevents bias | Promotes ethical development |
Quick Comparison
Principle | AI Transparency | AI Accountability |
---|---|---|
Key Components | - Model interpretability - Explainable AI - Transparent decision-making |
- Accountability frameworks - Ethics boards - Audits and testing - Human oversight |
Benefits | - Building trust - Improved decision-making - Reduced bias |
- Building trust - Improved decision-making - Reduced risk |
Challenges | - Complexity - Lack of standards - Data quality |
- Complexity - Lack of standards - Data quality |
Related video from YouTube
Understanding AI Transparency
AI transparency is about making the decisions of AI models clear and understandable. This helps ensure AI systems are fair, unbiased, and reliable, which is key to building trust in AI. It involves explaining how AI systems work, including how they process data, make decisions, or predict trends.
Key Principles of AI Transparency
Principle | Description |
---|---|
Explainability | Documenting how AI systems work and why they make certain decisions. This includes understanding the data used, the algorithms employed, and the decision-making processes. |
Accountability | Holding organizations and individuals responsible for the outcomes and impacts of AI systems. |
Benefits of AI Transparency
Benefit | Description |
---|---|
Building trust | Provides a clear understanding of how AI works and makes decisions. |
Preventing bias | Helps identify and prevent biases in AI systems. |
Improving reliability | Allows developers to identify and fix errors or flaws. |
Challenges of AI Transparency
Challenge | Description |
---|---|
Complexity | AI systems can be difficult to understand, making it hard to explain their decision-making processes. |
Trade-offs | Balancing explainability and AI performance can be challenging. |
Data quality | High-quality data is needed, which can be hard to obtain in some fields. |
Overall, AI transparency is crucial for responsible AI development and use. While it can be challenging, it helps build trust, prevent bias, and improve reliability.
Understanding AI Accountability
AI accountability means that people and organizations are responsible for the results and effects of AI systems. It ensures that AI systems are designed, built, and used in a way that is clear, understandable, and fair. Accountability is key in AI development and use, as it helps build trust, avoid bias, and improve reliability.
Key Components of AI Accountability
Component | Description |
---|---|
Transparency | Clear explanations of how AI systems work and make decisions. |
Explainability | Documenting the decision-making processes and algorithms used. |
Responsibility | Holding people and organizations accountable for AI outcomes. |
Auditability | Ensuring AI systems can be checked for bias, errors, and other issues. |
Benefits of AI Accountability
Benefit | Description |
---|---|
Building trust | Transparent and explainable AI systems build user trust. |
Preventing bias | Helps identify and avoid biases in AI systems. |
Improving reliability | Ensures AI systems are reliable and accurate. |
Challenges of AI Accountability
Challenge | Description |
---|---|
Complexity | AI systems can be complex, making accountability hard. |
Lack of standards | Few standards and regulations for AI accountability. |
Data quality | Poor data quality can hinder accountability in AI systems. |
Overall, AI accountability is key for responsible AI development and use. By ensuring transparency, explainability, and responsibility, organizations can build trust, avoid bias, and improve reliability in AI systems.
1. AI Transparency
Key Principles
AI transparency means making the decisions of AI systems clear and understandable. The main principles are:
- Understandability: AI should provide clear reasons for its decisions.
- Interpretability: AI models should be easy to understand.
- Explainability: AI should explain its decisions clearly.
Components
Component | Description |
---|---|
Model interpretability | Understanding how an AI model works and makes decisions. |
Explainable AI | AI's ability to provide clear reasons for its decisions. |
Transparent decision-making | Understanding how AI makes decisions and takes actions. |
Benefits
Benefit | Description |
---|---|
Building trust | Users trust AI systems that are clear about their decisions. |
Improved decision-making | Clear explanations help in making better decisions. |
Reduced bias | Transparency helps identify and reduce bias. |
Challenges
Challenge | Description |
---|---|
Complexity | AI systems can be hard to understand. |
Lack of standards | Few rules exist for AI transparency. |
Data quality | Poor data can make transparency difficult. |
2. AI Accountability
Key Principles
AI accountability means that people and organizations are responsible for the actions and decisions of their AI systems. The main principles are:
- Transparency: AI systems should clearly explain their decision-making processes.
- Responsibility: People and organizations should be accountable for AI actions and decisions.
- Explainability: AI systems should provide understandable reasons for their decisions.
Components
Component | Description |
---|---|
Accountability frameworks | Guidelines and policies for AI development and use. |
Ethics boards | Groups overseeing AI development and use. |
Audits and testing | Regular checks to ensure AI systems work as intended. |
Human oversight | Monitoring AI systems to prevent unintended harm. |
Benefits
Benefit | Description |
---|---|
Building trust | Helps build trust in AI systems and their decisions. |
Improved decision-making | Leads to better decisions and less bias. |
Reduced risk | Lowers the risk of unintended harm from AI systems. |
Challenges
Challenge | Description |
---|---|
Complexity | AI systems can be hard to understand, making accountability tough. |
Lack of standards | Few rules exist for AI accountability, making guidelines hard to set. |
Data quality | Poor data can make accountability difficult. |
Pros and Cons
AI Transparency Pros and Cons
Pros | Description |
---|---|
Builds Trust | Helps build trust in AI systems and their decisions. |
Improved Decision-Making | Leads to better decisions and less bias. |
Reduced Risk | Lowers the risk of unintended harm from AI systems. |
Detects Biases | Allows developers to identify biases and discriminatory patterns. |
Enhances Accuracy | Helps developers fine-tune algorithms and processes more effectively. |
Cons | Description |
---|---|
Vulnerability to Hacking | Explanations can be hacked, making AI more vulnerable to attacks. |
Increased Vulnerability | Disclosures can make companies more susceptible to lawsuits or regulatory action. |
Complexity | AI systems can be hard to understand, making transparency tough. |
Lack of Standards | Few rules exist for AI transparency, making guidelines hard to set. |
Data Quality | Poor data can make transparency difficult. |
Conclusion
AI transparency and accountability are key parts of responsible AI development and use. By making AI systems clear, understandable, and accountable, businesses can build trust, reduce risks, and ensure fair decisions. While there are challenges, such as securing data, explaining complex models, and keeping transparency with evolving AI, the benefits are greater.
As AI changes industries and our lives, it's important for everyone to focus on transparency and accountability. This way, we can get the most out of AI while keeping risks low. We encourage businesses, policymakers, and AI developers to work together to set standards and best practices for AI transparency and accountability, making sure AI benefits everyone.
FAQs
What are the requirements for transparency in AI?
There are three key requirements for transparent AI: explainability, interpretability, and accountability.
Requirement | Description |
---|---|
Explainability | AI should provide easy-to-understand reasons for its decisions and actions. |
Interpretability | People should be able to understand how an AI model works and behaves. |
Accountability | AI systems should be held responsible for their actions and decisions. |
These requirements apply to training data, algorithms, and decision-making in AI systems.
Ready to Transform Your Phone System?
See how Dialzara's AI receptionist can help your business never miss another call.