Federated Learning for Customer Service AI
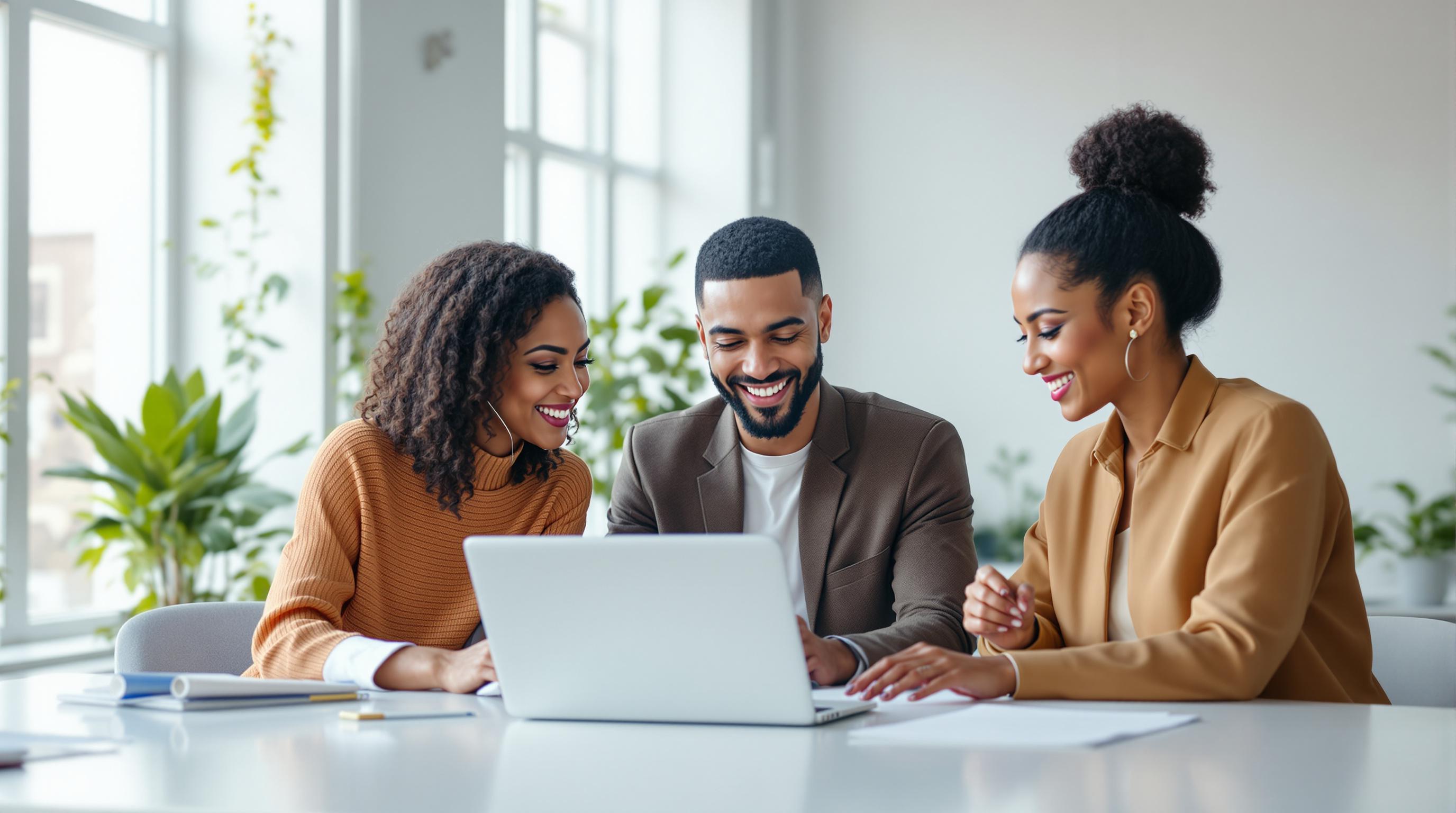
Explore how federated learning enhances customer service AI by prioritizing privacy, personalization, and operational efficiency.
Federated learning is reshaping customer service by enabling AI to improve without compromising privacy. Here’s a quick overview of its key benefits:
- Privacy First: Data stays local, ensuring compliance with regulations like GDPR and CCPA.
- Personalized Service: AI adapts to specific customer needs, such as regional accents or industry-specific language.
- Scalability: Businesses can handle higher call volumes without major infrastructure changes.
- Security: Decentralized systems reduce the risk of data breaches.
For example, Dialzara’s AI system uses federated learning to deliver secure, efficient, and tailored customer interactions. This approach helps businesses meet customer expectations while protecting sensitive information.
Main Advantages in Customer Service
This section delves into how federated learning improves customer service by addressing privacy, personalization, and operational efficiency.
Data Privacy and Regulation Compliance
Federated learning processes data locally, keeping sensitive information secure while improving AI functionality. This method aligns with regulations like GDPR and CCPA by ensuring that only insights - not raw data - are shared. For example, Dialzara's AI system uses this approach to maintain privacy while delivering effective solutions. This setup also allows for personalized customer interactions, which we’ll explore further.
Customer-Specific Service Delivery
By processing data locally, AI systems can better understand and cater to specific customer needs. Instead of relying on generic models, the AI adjusts to the unique features of each business.
Dialzara's AI phone system, for instance, adapts to:
- Industry-specific language
- Regional accents and phrases
- Patterns in customer inquiries
- Communication styles unique to each business
This fine-tuned understanding helps meet local customer expectations without risking privacy.
Resource Management and Growth
Federated learning offers operational advantages that support business growth:
- Reduced Data Transfer: Only model updates are shared, minimizing bandwidth use.
- Efficient Storage: Removes the need for large central data centers.
- Rapid Deployment: Quick setup without heavy infrastructure requirements.
This decentralized approach ensures businesses can scale their customer service operations while maintaining high-quality interactions. Even as call volumes grow, service levels stay consistent without requiring significant infrastructure upgrades.
Setting Up Federated Learning Systems
Implementation Steps
Here's how to set up federated learning for customer service AI, ensuring data protection while learning from distributed interactions:
-
Initial System Configuration
Start by setting up a secure business profile through Dialzara. -
Building the Knowledge Base
Upload key training materials to create a strong knowledge foundation for your AI. -
Local Training Setup
Adjust the system to handle interactions locally, ensuring sensitive data stays secure. Choose a voice that aligns with your brand's tone, then connect your system to live communication channels. -
Integration and Testing
Link the system to your communication channels using call forwarding. Test the AI's performance and verify that privacy protocols are functioning as expected.
Problem-Solving Guide
Once the basic setup is complete, use these steps to tackle challenges. Start with a trial phase to monitor performance and resolve any early issues before rolling out the system fully.
Current Applications
AI Agent Improvements
Federated learning is changing how AI-powered customer service agents evolve, all while keeping data privacy intact. This approach allows models to learn from decentralized interactions without exposing sensitive information. For example, Dialzara's AI phone answering service uses federated learning to improve response accuracy and create natural conversations - all without compromising customer data or violating privacy rules.
By processing data locally, the system identifies key learning patterns while keeping personal information secure. This has led to advancements in:
- Context understanding and personalization: Recognizing industry-specific terms and aligning with business communication styles.
- Task accuracy: Improving precision in tasks like scheduling appointments and relaying messages.
- Privacy protection: Safeguarding sensitive data throughout the process.
These updates not only enhance functionality but also reinforce strong security practices.
Security and Risk Management
Beyond improving AI agents, federated learning strengthens system security. By combining local data processing with encrypted updates, the risk of breaches is significantly reduced. Key advantages include:
- Decentralized processing with secure, encrypted learning updates.
- Improved fraud detection capabilities.
- Compliance with data protection regulations.
- Reduced risks of unauthorized access.
Sector-Specific Examples
The benefits of federated learning extend across various industries, transforming customer service and security standards.
Healthcare
- Safely managing patient inquiries.
- HIPAA-compliant appointment scheduling.
- Securely exchanging medical information.
Financial Services
- Verifying transactions securely.
- Handling account inquiries privately.
- Ensuring compliance in customer authentication.
Real Estate
- Protecting property information.
- Securely scheduling appointments.
- Keeping client data confidential.
These examples highlight how federated learning helps businesses deliver efficient, secure customer service while adhering to strict privacy standards.
Next Steps in Development
Privacy Protection Updates
Upcoming updates in federated learning aim to boost data privacy by processing information directly on local devices while still improving AI models. Dialzara's system is a great example, as it processes data locally and uses secure, aggregated updates to refine its models. These privacy-focused approaches are paving the way for new advancements in AI-powered customer service.
New Technology Connections
New technologies working alongside federated learning are opening doors for better customer service solutions. As businesses grow and strive to keep interactions personalized, effective AI tools are becoming more important than ever.
Here’s an interesting stat: 60% of customers prefer local businesses, yet only 38% of calls are answered, and just 20% of callers leave a voicemail. This gap highlights the need for advanced AI systems. The next wave of AI will offer real-time processing and more accurate responses tailored to customer needs.
Client feedback consistently points to the system's reliability and ability to make effective decisions. These advancements are helping businesses handle larger call volumes without sacrificing service quality or privacy protections.
Summary
Main Points
Federated learning is changing AI customer service by focusing on privacy and delivering more tailored, efficient interactions. A great example of this is Dialzara's AI virtual receptionist, which balances privacy with personalized experiences.
Here’s a key insight: 60% of customers prefer local calls, but only 38% actually get answered[1]. This gap highlights the demand for secure and scalable AI solutions.
Key advantages include:
- Better Privacy: Data is processed locally, which helps improve models without compromising security.
- Stronger Personalization: Customizes services to fit unique business needs while keeping data safe.
- Scalability: Handles growing call volumes without sacrificing service quality.
This demonstrates how federated learning consistently delivers reliable performance.
Together, these benefits help businesses handle increasing service demands, maintain 24/7 availability, and stay compliant with regulations - all while offering secure and scalable AI-driven solutions.
Ready to Transform Your Phone System?
See how Dialzara's AI receptionist can help your business never miss another call.